To Trust or to Stockpile
Modeling Human-Simulation Interaction in Supply Chain Shortages
Motivation
The COVID-19 pandemic, in its early days, provoked panic-buying and hoarding behavior in millions of people to the extent that caused a toilet paper shortage across many countries. The same type of behavior can disrupt the supply of many critical products including pharmaceutical drugs. Therefore, it is essential to study the role of human behavior in critical systems and especially in the presence of adverse events such as natural disasters, health crises, and financial crises.
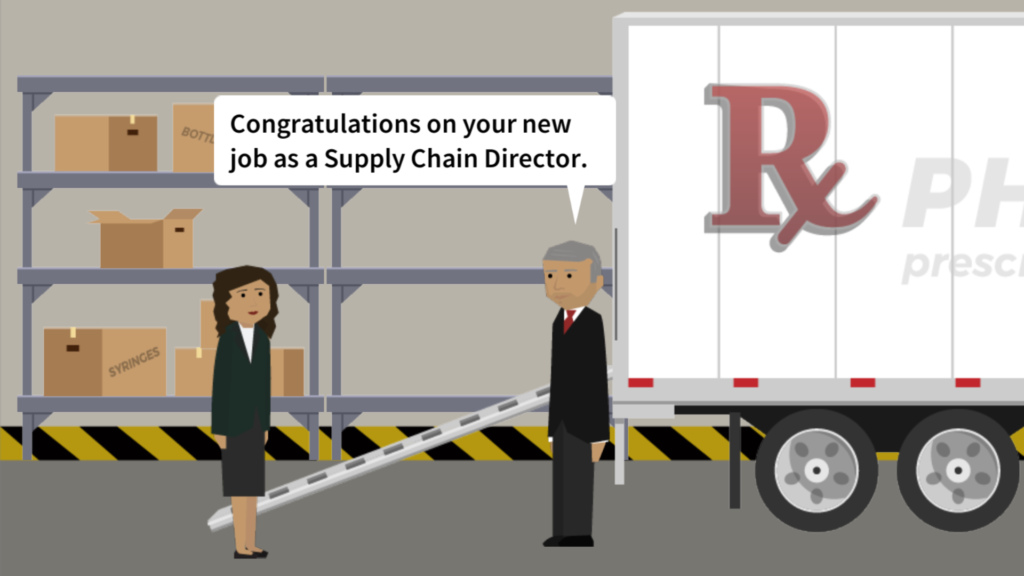
Game-Based Simulations
For years researchers have been using simulations to study human behavior. In the past decade, game-based simulation environments such as Gamettes are increasingly used to conduct behavioral experiments and advance our understanding of human behavior and decision-making. See Gaming Simulation or Participatory Simulation approaches as other examples of such environments. Read More…
The dynamic state of the simulation makes it challenging to model human behavior.
The system’s state affects humans’ behavior, but it can also dynamically change due to human interaction with the simulation. Besides, researchers often use game-based simulations to generate hypotheses and test them; hence, they create manipulations that may have unexpected effects on human behavior.
Our Goal is to Characterize Human-Simulation Interaction
We characterize Human-Simulation Interaction by:
1. Characterizing the System's State
We characterize the system’s states by reducing the dimensionality of the state space of the simulation using Principal Component Analysis (PCA) and performing hierarchical cluster analysis on component scores to find similar states.
2. Characterizing the Behavior of Players
We use Hidden Markov Models (HMMs) to find players’ response modes in their sequential decisions. We also make player profiles and find different player types by analyzing the sequences of players’ response modes.
3. Characterizing the Interaction
We characterize the interaction by analyzing the sequences of players’ response modes across the system’s states and showing how the behavior changes in different states. Through this characterization, we also test the effect of experimental manipulation.
Gamette Experiment
We recruited 121 online participants through the Prolific app and asked them to play a Gamette replicating supply chain decisions. The players played the role of a wholesaler in a supply chain consisting of two manufacturers, two wholesalers, and two health centers in a pharmaceutical supply chain. The task of each player was to order from their supplier and allocate drugs to health centers for 35 game weeks. They all received order suggestions (where they could follow or adjust) and experienced a manufacturing disruption on Week 28.
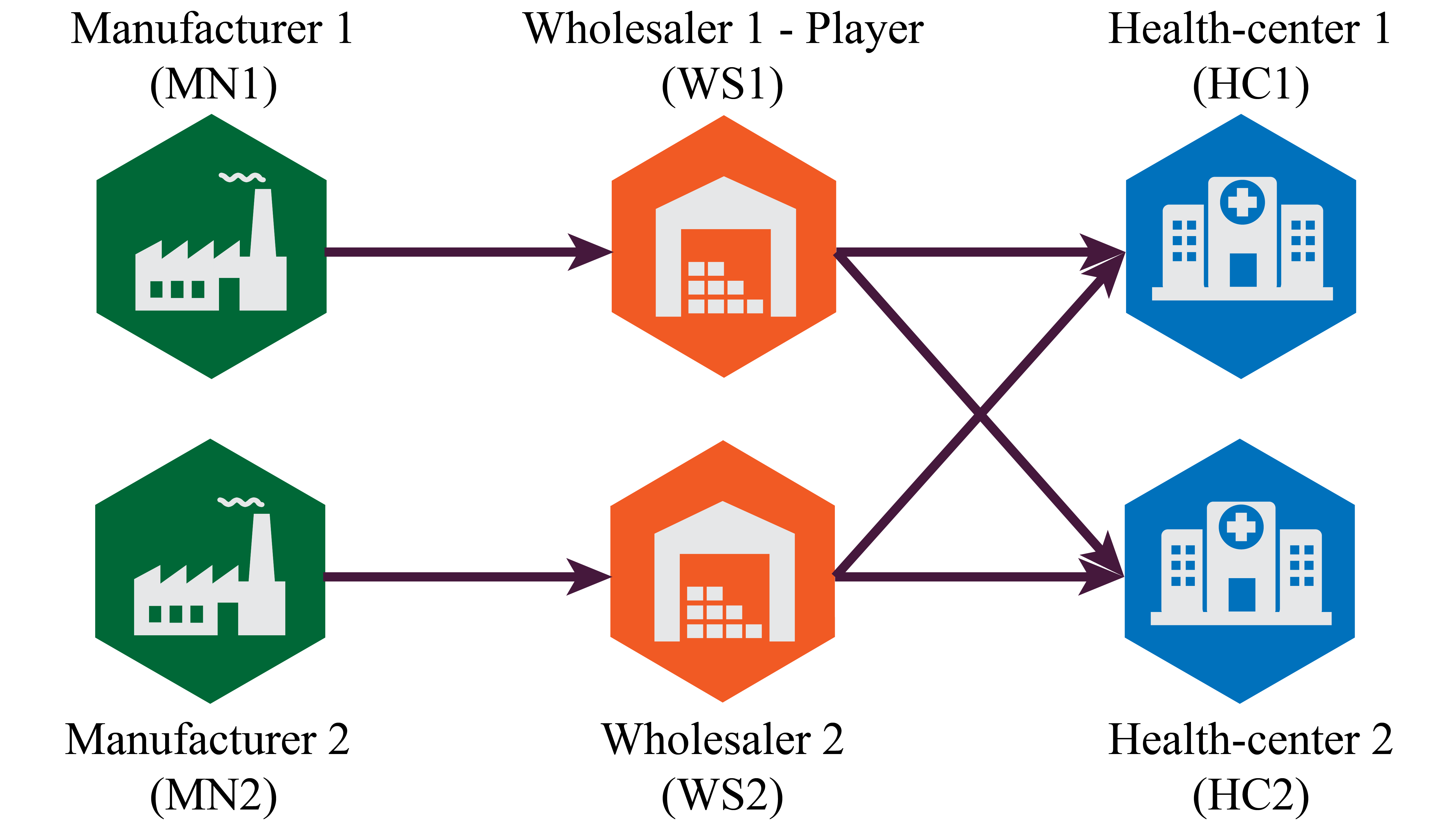
We also created a manipulation where for some players we shared information on their supplier inventory and for others, we did not.
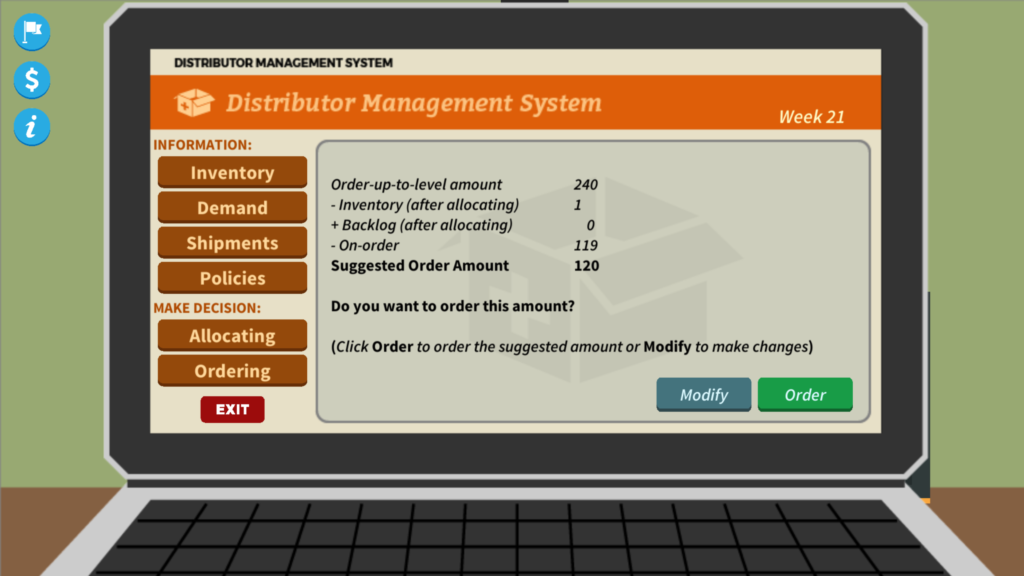
The ordering scene without information sharing
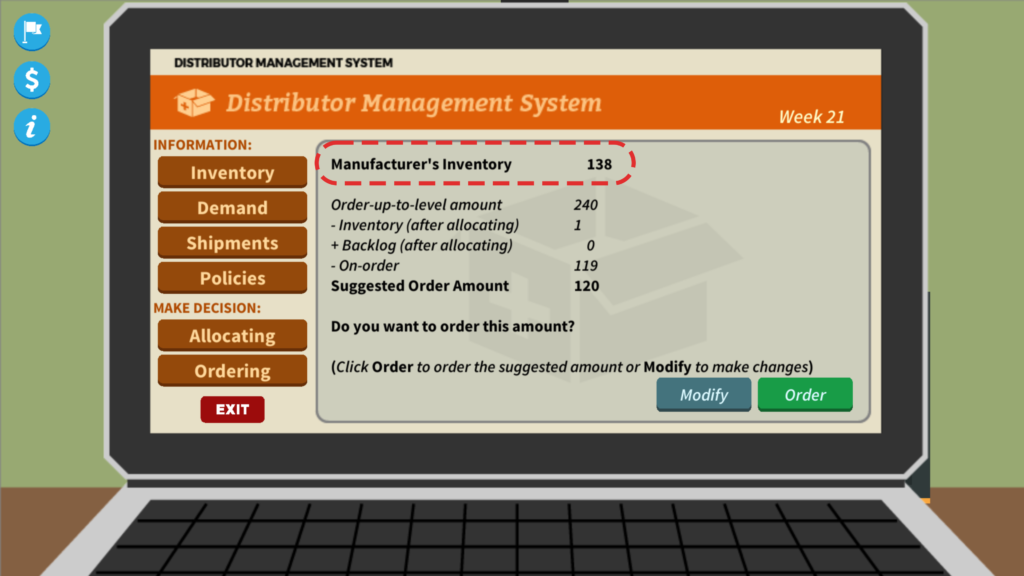
The ordering scene with information sharing
1. Characterizing the System
We applied PCA to reduce the dimensionality of the state space including inventory, demand from each health center, backlog, received-shipment, and on-order for all players and across the 35 game-weeks they played. We found that two principal components explain 77% of the variance in data. Component 1 was highly correlated with an increase in backlog and on-order. Therefore, we considered it to be a measure of supply-side disruption. Component 2 was highly correlated with an increase in inventory and received shipments from the supplier; hence, we considered Component 2 to be a measure of recovery after disruption where participants start to receive large shipments after a period of shortage. Next, we computed the scores for each principal component and performed a hierarchical cluster analysis where we identified three distinct system states.
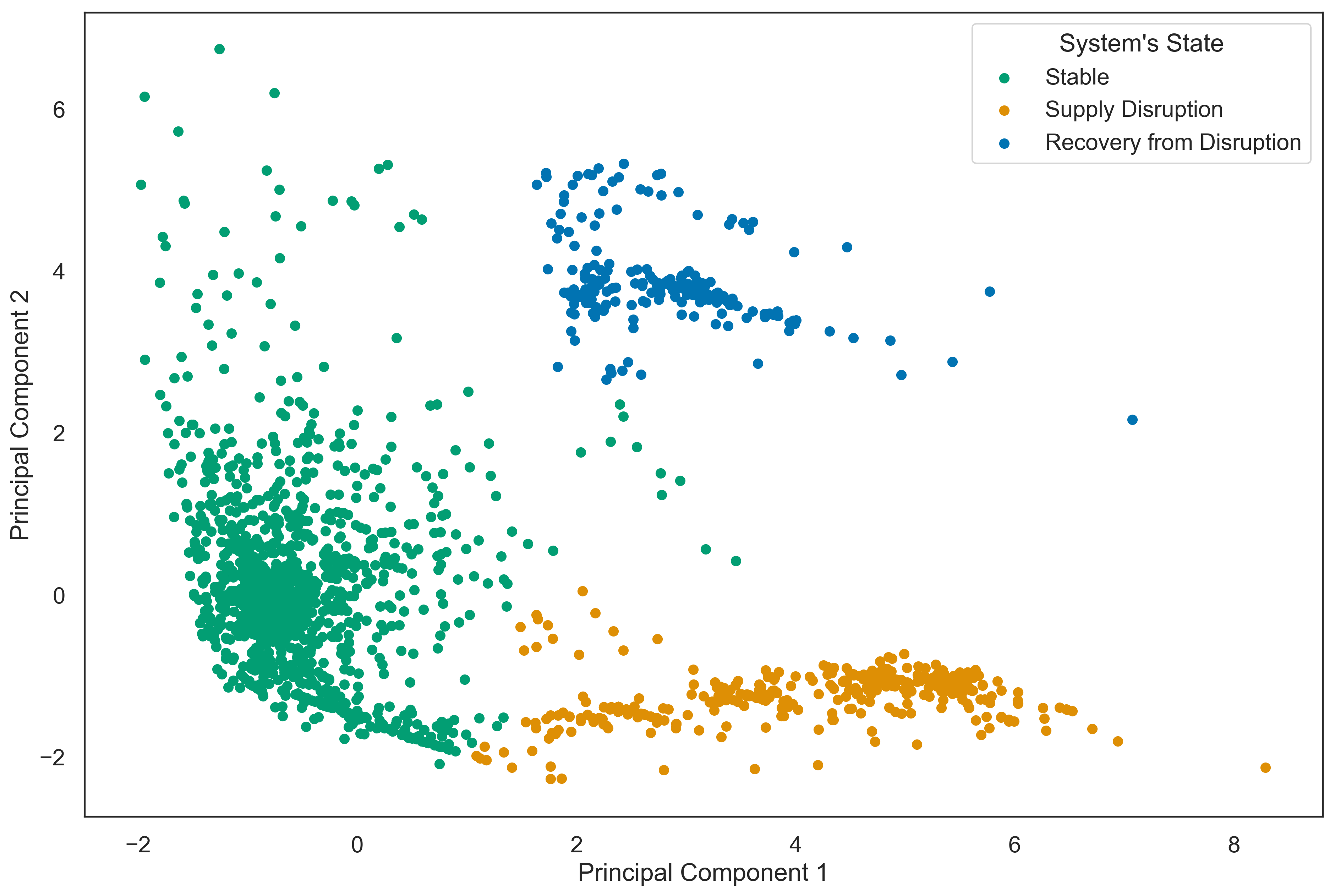
Principal components and their scores and the emerged system’s states.
Stable
The Stable state represents the situation where players consistently receive shipments from the supplier and are able to satisfy the demand from health centers.
Supply Disruption
The Supply Disruption state corresponds to an increase in backlog and on-order amounts and represents a situation where players do not receive enough shipments to satisfy their demands.
Recovery from Disruption
The Recovery from Shortage state corresponds to an increase in Component 2 scores, suggesting an increase in received shipments and inventory.
We also counted the number of players in each state over time and used the majority of players in each state to divide the simulation into five phases. In Phase 1, the system is in a stable state. In Phase 2, the system is still in a stable state, however, players are notified about manufacturing disruption. In Phase 3, most players experience a shortage by receiving fewer shipments from the supplier and incurring high backlog and on-orders. In Phase 4, all players receive large shipments from their recovered supplier. In Phase 5, the majority of players move back to a stable state.
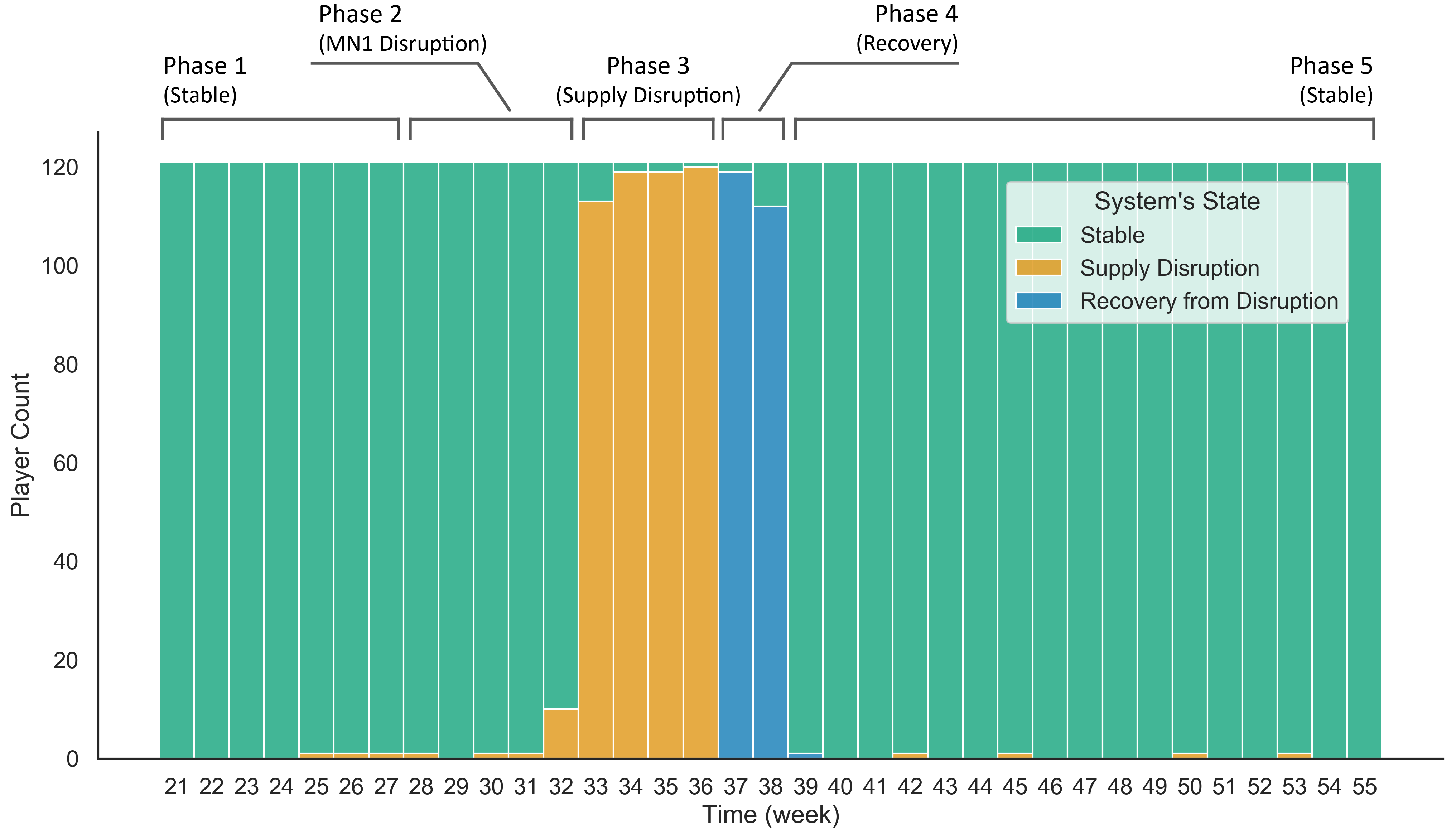
Count of players in each system’s states and the corresponding system phases based on PCA cluster analysis. Each phase represents a specific state. Note that Phase 2 starts with Week 28 which is when MN1 is disrupted.
2. Characterizing the Behavior
We modeled an HMM with eight hidden states using the normalized deviations from the suggested order amounts as the observation sequences, where each hidden state represents a behavioral response mode. According to this model, we found two modes of negative adjustment (N1 and N2), a control mode (C), and five modes of positive adjustment (P1, P2, P3, P4, P5).
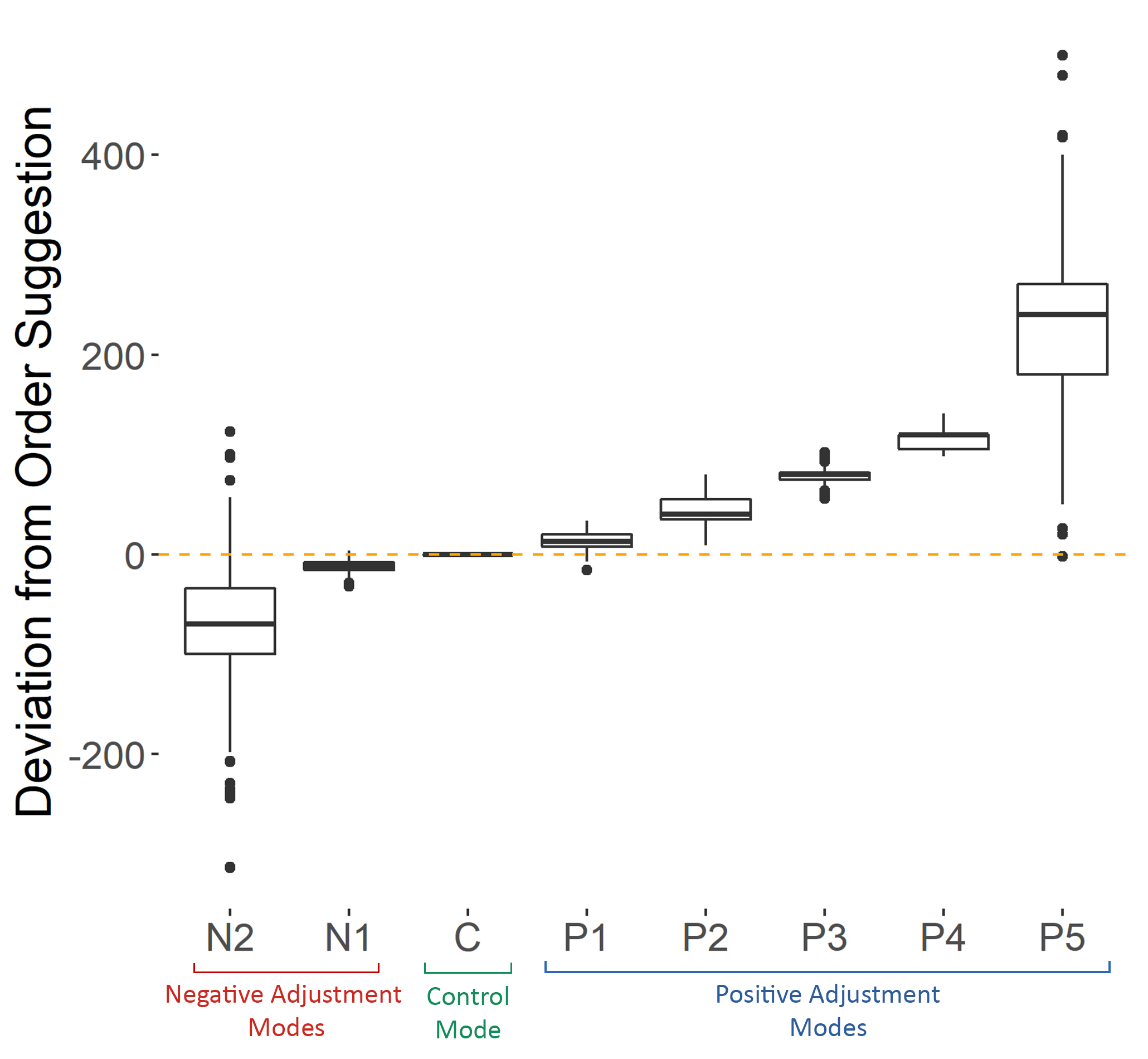
HMM emission distributions for deviations from order suggestions in each hidden state (i.e., response mode). Each response mode corresponds to a certain level deviation from order suggestions.
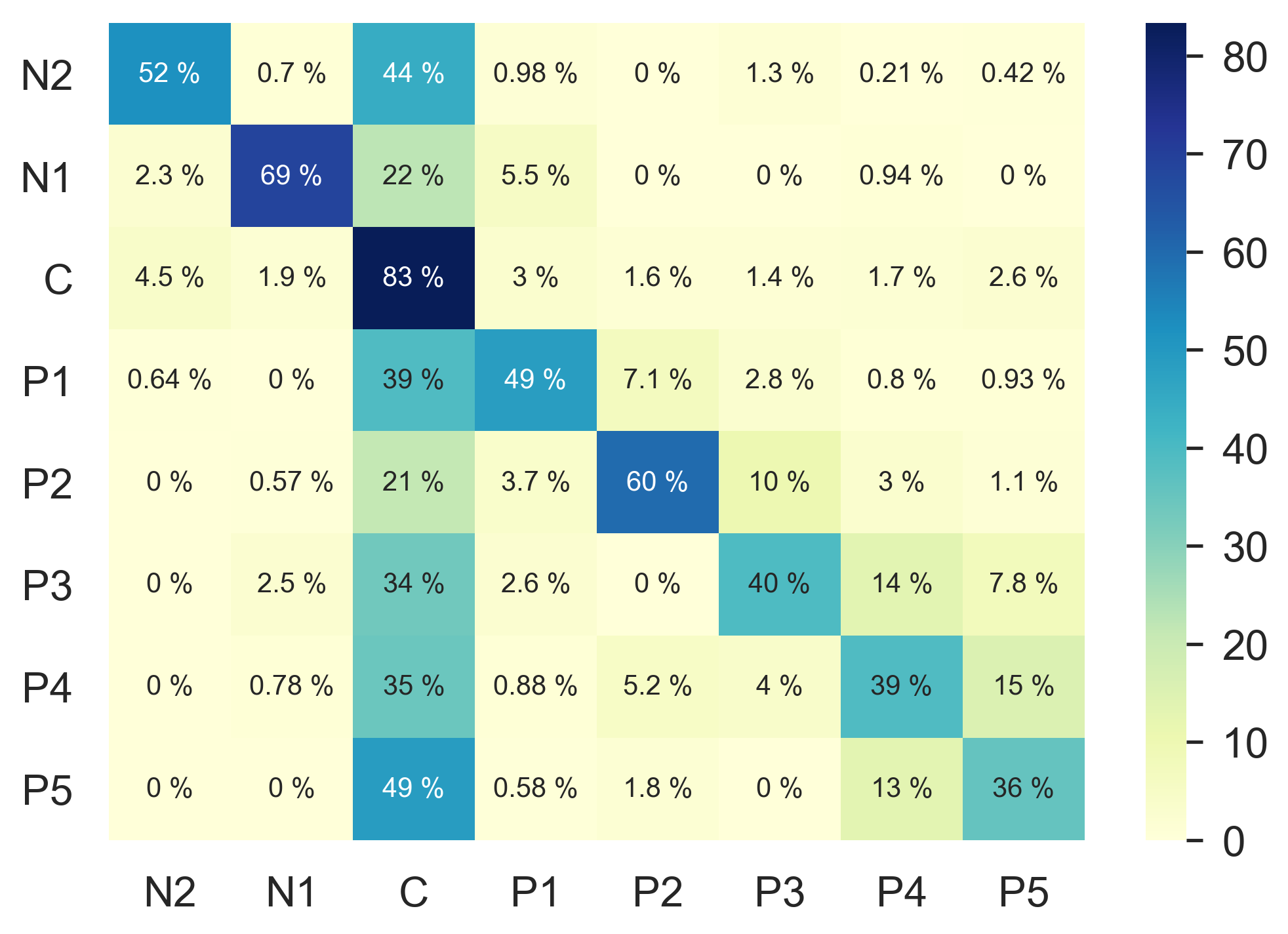
Transition rates of the HMM hidden states (i.e., response modes) for all players. Each response mode corresponds to a certain level deviation from order suggestions.
We also obtained the sequences of response modes for each player and used these sequences to find distinct player types by performing hierarchical cluster analysis. We found three types of players with distinct decision patterns.

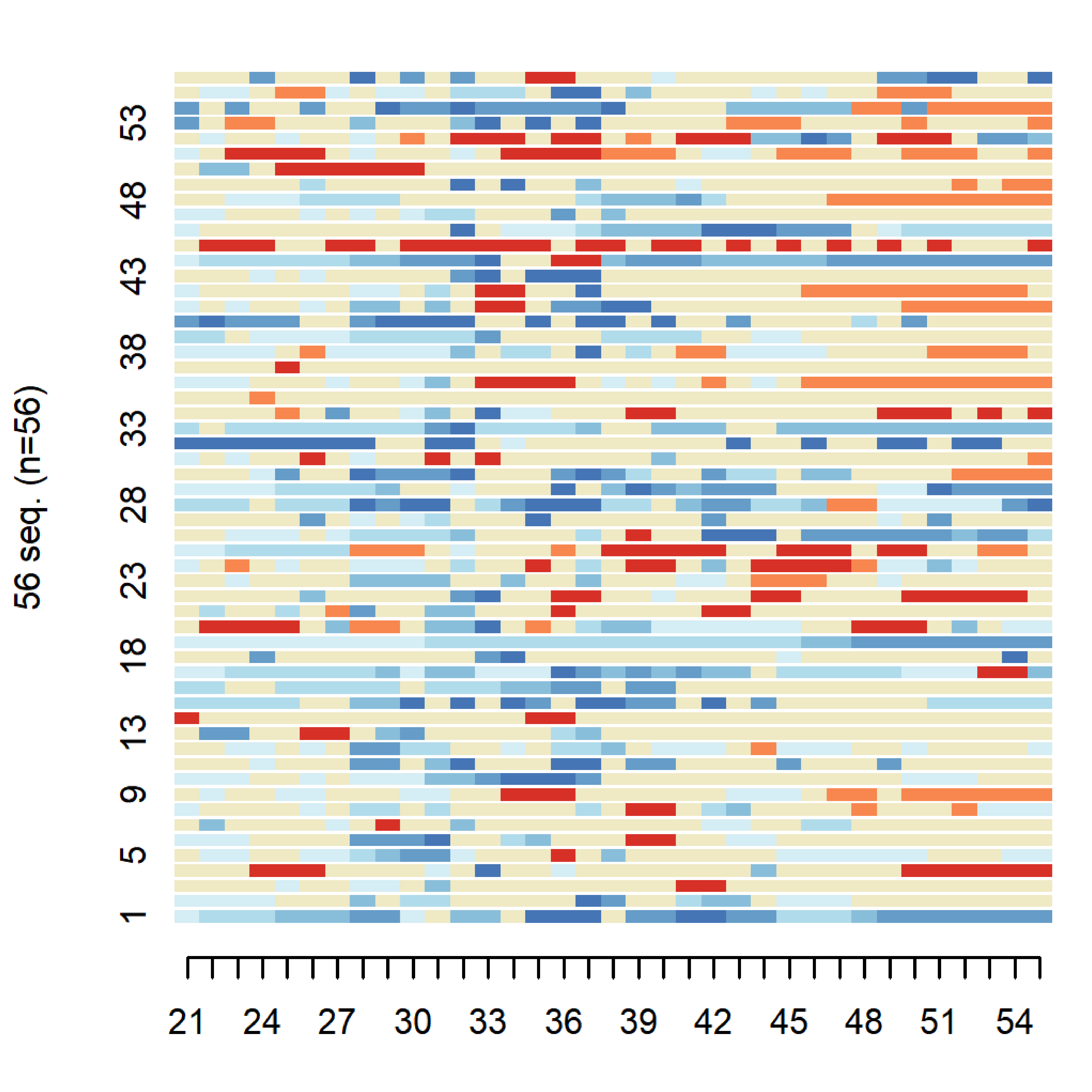
Hoarders
Hoarders stay in or transit to other positive adjustment modes with a higher probability compared to reactors. They tend to “ignore order suggestions”, “adjust order suggestions”, and their strategy is to “stockpile”.
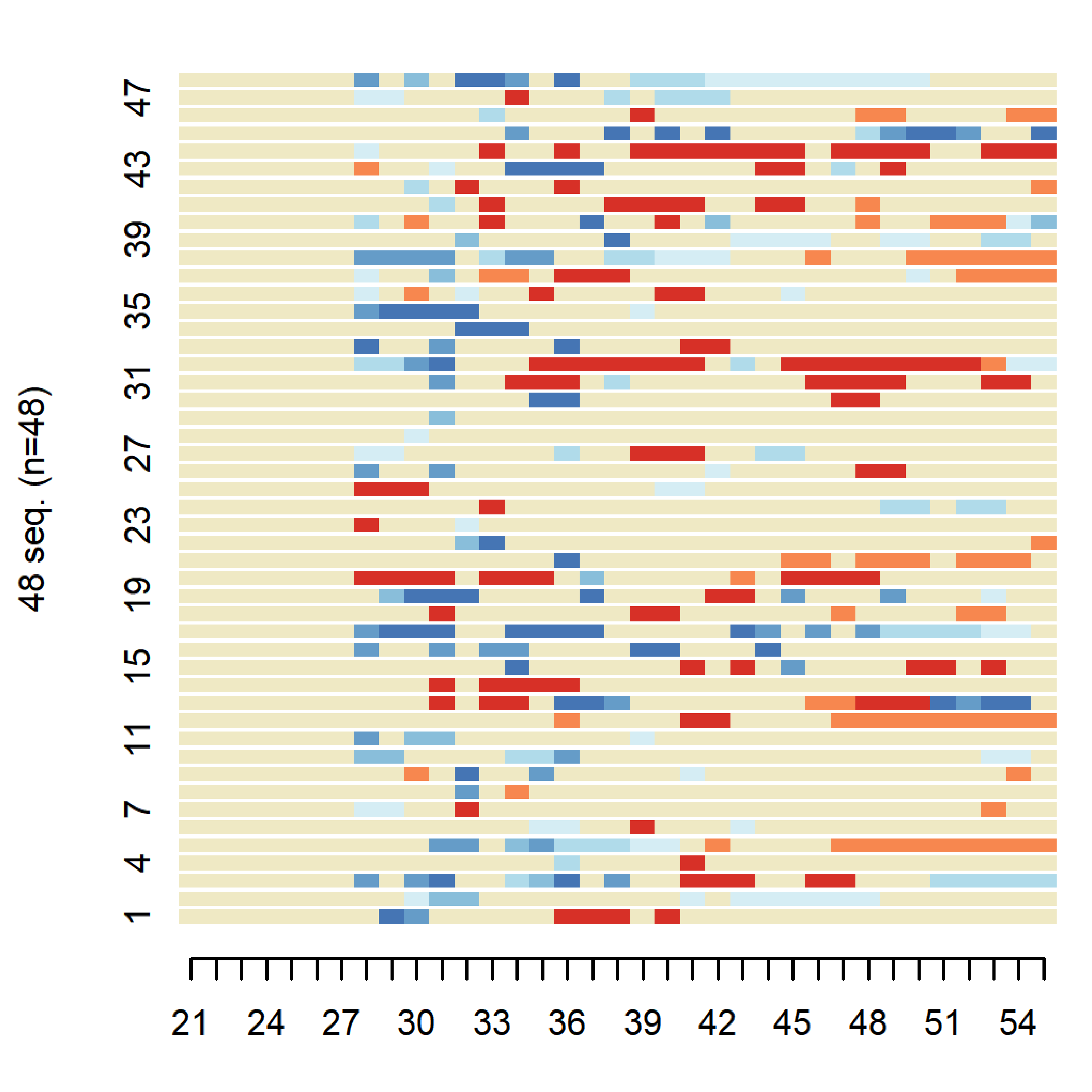
Reactors
Reactors start the game in control mode (C) by following order suggestions and stay in control mode until Week 28 where they react to the news about the disruption. Their strategy is to “keep safety stock” or to “stockpile” after the disruption.
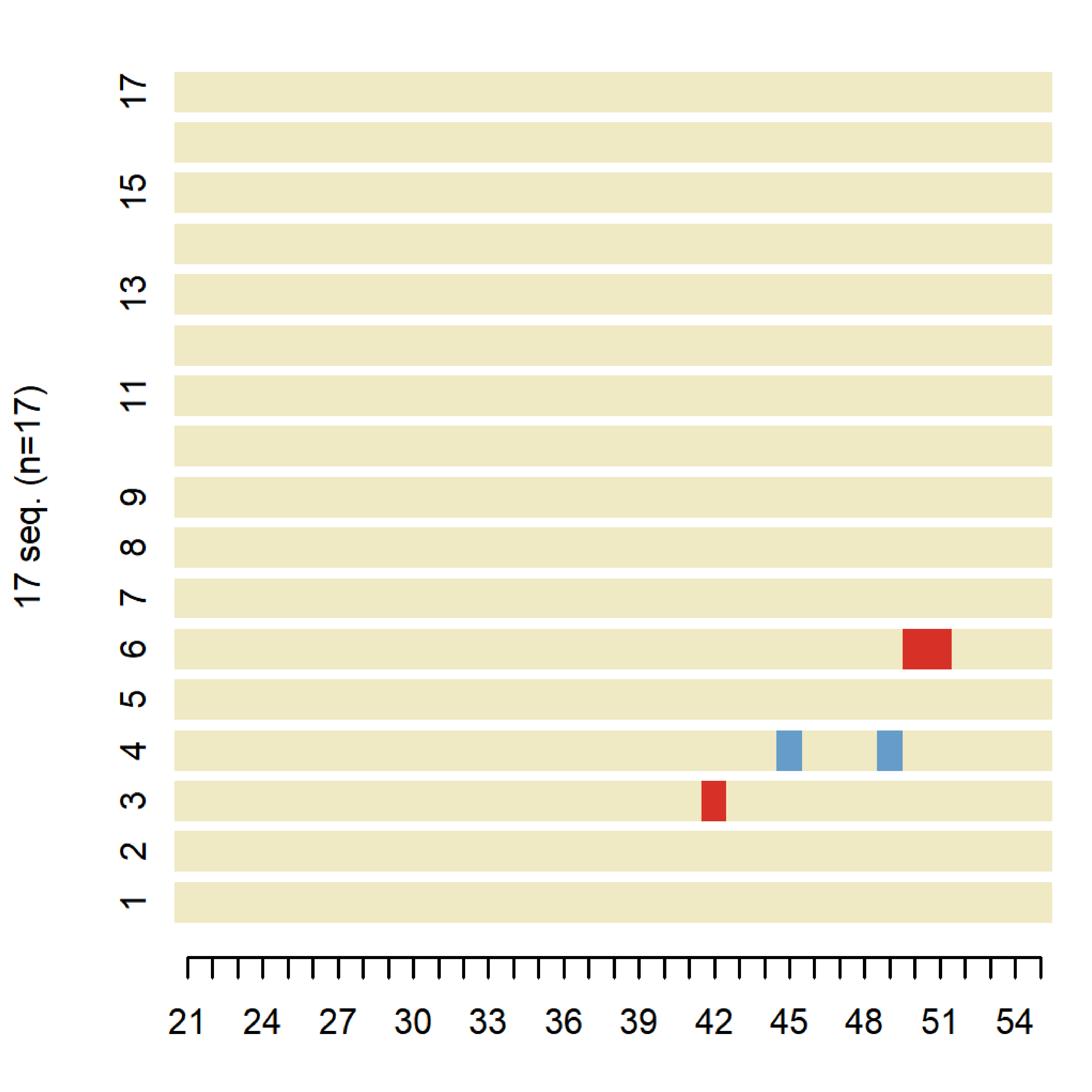
Followers
Followers consistently follow the order suggestions throughout the game and stay in the control mode with the highest probability compared to the other player types. Their strategy is to follow order suggestions.
3. Characterizing the Interaction
To characterize the interaction of players with the simulation, we focused on the behavioral response modes in each phase of the system to see how the system’s states, the player types, and our manipulation (information sharing vs. no information sharing) affect the interaction of players with the system.
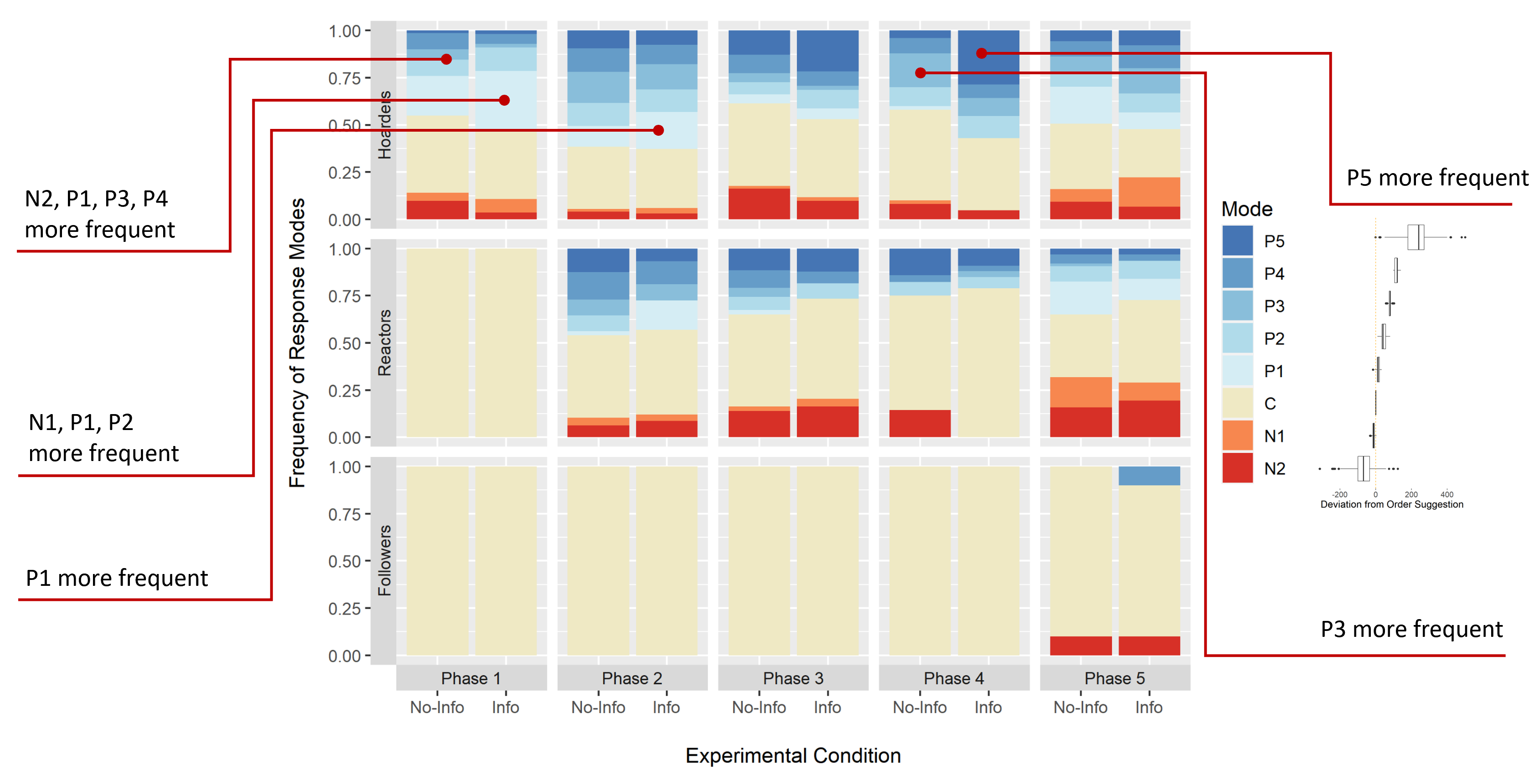
Frequency of players who contained each response mode in their sequences across system phase and experimental conditions.
The impact of the System
The system’s state affects human behavior. We see how Hoarders move to positive or negative adjustment modes depending on the state of the system, and how Reactors react to disruption in phase 2.
The Impact of Behavior
Not all humans adjust order suggestions the same way. We see Hoarders are more frequently in positive adjustment modes, reactors only adjust when facing uncertainties, and followers always follow suggestions.
The impact of Information Sharing
Information sharing helps reduce the deviations from order suggestions for Hoarders and in phases 1 and 2. However, it causes over-ordering in hoarders after the shortage period is over!
Main Takeaways
- People deviate more from the order suggestions when facing uncertainties such as a shortage. However, humans behave differently! Not all people respond in the same way to uncertainties. Some people (Followers) always follow recommendations regardless of the system’s state.
- Information sharing reduces the amounts of deviations from order suggestions. However, the state of the system and different behavioral types can affect how people use information sharing. We saw that Hoarders who received information sharing used this information to over-order and hoard even more after the shortage period was over. This finding challenges previous research on information sharing!
- Simulations are dynamic systems and it is important to analyze behavior by characterizing the human-simulation interaction.
Contribute to our research!
We are trying to understand how drug delivery supply chains can be more resilient.
Play our new Gamette and help us to learn more about human behavior within drug delivery supply chains.